当社は産業用ドローン向けに単眼カメラによる距離推定と物体検出のAI認識モデルを開発しました。
この動画は、実際にお客様のドローンでフィールドテストをした時のものです。動作環境としてはNVIDIA社Xavierを活用しています。
当社が開発したAI認識モデルは、ドローンが障害物にぶつからないようにする目的で使用しています。AI認識モデルは、ドローンの離陸時に前方の物体と物体までの距離を検出し、またドローンの飛行時は前方および前方下部の物体と物体までの距離を検出します。動画をご覧いただくとBounding Boxで物体検出「例: power_tower (送電塔)、person、turbine (風力発電用タービン)」し、Bounding Boxの下部にドローンからその物体までの距離を検出していることがおわかりになるかと思います。ドローンが離陸する際のカメラの角度による物体の見え方に関係なく、AI認識モデルは物体の種類を判別し距離を測ることが可能です。
今回のAI認識モデルの特徴は単眼カメラで距離推定を行っている点です。距離の推定には、従来からのオーソドックスな手法としてステレオカメラを用いる手法があります。この場合、事前のカメラキャリブレーションが必要であったり、コストやサイズの制約がありました。今回のAI認識モデルでは単眼カメラによる距離推定を行っており、物理的にカメラ1台で済むため省スペース化やコスト低減が期待できますし、事前のカメラキャリブレーションも一切不要です。単純に動画さえあれば、画素単位のち密な距離画像とカメラの移動軌跡を得ることができます。加えて、下記の画像で分かる通り、単眼カメラによる距離推定の他社製AIネットワークと比較しても、高精度な距離(深度)推定を実現することが可能です。さらに、車、ボート、鉄塔、家などの物体を認識するモデルと組み合わせることで、認識した特定の物体までの距離を推定しています。
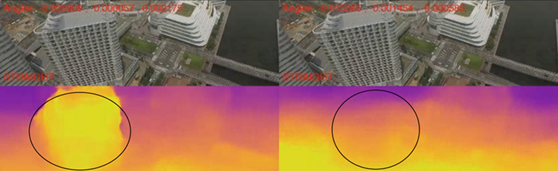
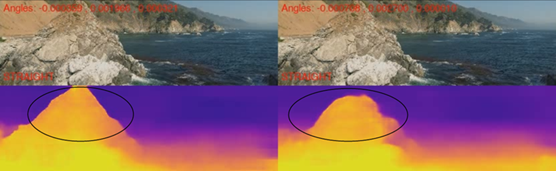
Comparison of accuracy of distance estimation: Left photos(DMP), Right photos (Another)
今回の産業用ドローンへの適用はお見せできる一例となりますが、距離推定は、SLAM技術と共に、自動運転車、工場や倉庫におけるAGV、UGV、お掃除ロボットなど様々なアプリケーションにおいてロボットの眼を実現する上での基盤技術です。当社は、現在ロボティクス分野の自律化・省人化に向けた技術開発に注力しており、SLAM技術や距離推定のAI認識モデルといった先進テクノロジーをもって、お客様の製品の自律・自動化に貢献していきたいと考えています。
We have developed an AI recognition model for industrial drones for distance estimation and object detection using a monocular camera.
This video shows an actual field test with a customer’s drone, in which NVIDIA Xavier was used as the operating environment.
The AI recognition model we developed is used to prevent the drone from hitting obstacles, which detects objects ahead of the drone and the distance to them when the drone takes off, and detects objects ahead of and below the drone and the distance to them when the drone is in flight. In the video, you can see that the Bounding Box detects objects, such as power_tower, person, and turbine, and the distance from the drone to the object at the bottom of the Bounding Box. Regardless of the angle of the camera at which the drone takes off, the AI recognition model is able to determine the type of object and measure the distance.
The feature of this AI recognition model is that it uses a monocular camera for distance estimation. The conventional orthodox method for distance estimation is to use a stereo camera. In this case, prior camera calibration is required and there are cost and size restraints. Our AI recognition model uses a monocular camera for distance estimation, which physically requires only one camera, thus saves space and reduces cost, and does not require any prior camera calibration. As long as you have a simple video, you can get pixel-perfect distance images and camera movement trajectories. In addition, as you can see in the image, it is possible to achieve highly accurate distance (depth) estimation compared to other AI networks that also use a monocular camera for distance estimation. Furthermore, combining it with the model that recognizes various objects such as cars, boats, pylons, and houses, you can estimate the distance to a specific object recognized.
This application to an industrial drone is just one example we can show, but distance estimation, together with SLAM technology, is a fundamental technology for realizing the robot’s eye in various applications such as self-driving cars, AGVs in factories and warehouses, UGVs, and cleaning robots. We are currently focusing on the development of technologies for autonomy and manpower saving in the field of robotics, and will contribute to the autonomy and automation of our customers’ robotic products with our advanced technologies such as SLAM technology and AI recognition models for distance estimation.