この度、株式会社リョーサン(以下、リョーサン)が二子玉川の蔦屋家電+に出展している協働型ロボット※デモに周辺監視のエッジAI画像認識ソフトウエアを提供しました。
※協業(型)ロボット:産業用ロボットの一種で、人との共同作業を前提としたロボット。人の接近を感知したら自動的に停止するといった機能を備える
DMPが提供したAI認識ソフトウエアは、協働ロボットの安全操作をサポートするものです。ロボット上部のカメラ映像から周辺の障害物を検出してロボットに通知し、障害物が検出されるとロボットは減速して安全性を確保します。10月25日まで蔦屋家電+にてデモ展示していますので、お近くにお越しの際は是非足をお運びください!
https://store.tsite.jp/tsutayaelectricsplus-futako/
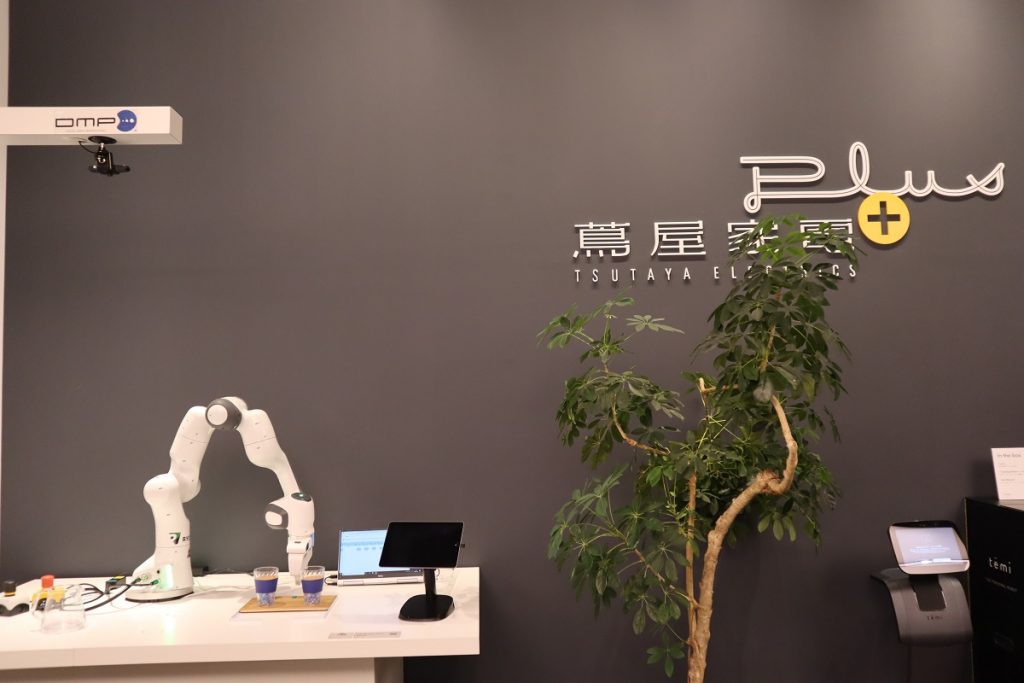
さて、ここからは今回技術面で工夫したお話を少しさせてください。
もともとDMPは周辺監視を目的としたAI認識ソフトウエアを持っていましたが、リョーサン様からご要望いただいた蔦屋家電+での出展協力の内容に合わせていくつか工夫をしました。
工夫した内容
1) 障害物の定義
もとのAI認識ソフトウエアは工場内のロボットや特定設備の周辺監視を目的にしていました。しかし、今回の設置場所である蔦屋家電+には多くの方がご来場されるため、工場内とは異なり、例えば子供のおもちゃが飛んでくるといった事前に予想できない様々な障害物が考えられます。そのため、特定の物体を検出する方法ではなく、映像を時系列に比較し、差分が発生した場合に、障害物アリと判断するような仕様に工夫しました。
2) 検出範囲と検出感度
物体認識の検出範囲はカメラの取付位置やロボットの姿勢によって大きく変動します。ロボットは一定の姿勢ではなく動作をしますので、物体のカメラからの見え方が絶えず変わります。事前に蔦屋家電+の現場やロボットの動作内容が不明だったため、デモ当日にカメラの設置位置やリョーサン様のデモ内容に応じて柔軟に検出範囲を設定できるように工夫しました。また、検出感度に関しては、過剰に検出しても、またしなさ過ぎてもシステムとして成立しません。過去の画像と比較する際のパラメータを複数作り、適切な感度に調整できるように工夫しました。
3) AI認識モデルの軽量化
もとのAI認識モデルはGPUで動作することを前提に作っていました。GPUは高性能な反面、高価でもあります。今回のデモシステムを多くのお客様にご提供していく上で安価である必要があります。そのため、今回はGPUではなくラズパイ(Raspberry Pi)を採用しました。ただし、ラズパイはGPUほど性能を持っていませんので、AI認識モデルを軽量化する工夫をしました。
感染症拡大や人手不足といった社会課題の解決に向けて、協働ロボットの需要は伸びており、世界市場が年率40%以上で成長するという予測もあります。当社は、ロボティックビークルを含むロボットのAI化に注力し、社会課題の解決に貢献してまいります。
We provided the edge AI image recognition software for peripheral monitoring for Ryosan’s demonstration of the collaborative robot * exhibited at TSUTAYA ELECTRICS + (PLUS) in Futako-tamagawa, Tokyo.
* Collaborative robot: A type of industrial robot that is premised on collaborative work with humans. It has functions such as to automatically stop when it detects the approach of a human.
The AI recognition software provided supports the safe operation of collaborative robots. Obstacles in the vicinity are detected from the image of camera on the top of the robot and notified to the robot, and when an obstacle is detected, the robot slows down to ensure safety. This exhibition will last until October 25th, so please stop by when you are around!
https://store.tsite.jp/tsutayaelectricsplus-futako/english/
Now, let us explain a little bit about the technical ideas this time.
We already have AI recognition software for the purpose of peripheral monitoring, but we made some ingenuity according to the content of the support requested by Ryosan.
Ingenious points
1) Definition of obstacles
Our AI recognition software is originally intended to monitor the surroundings of robots and specific equipment in factories. However, since many people come to TSUTAYA ELECTRICS +, which is the installation location this time, there are various obstacles that cannot be predicted in advance, such as children’s toys coming flying, unlike in the factory. Therefore, instead of detecting a specific object, we have devised a specification that compares images in chronological order and determines that there is an obstacle when a difference in image is detected.
2) Detection range and detection sensitivity
The detection range of object recognition varies greatly depending on the mounting position of the camera and the posture of the robot. Since the robot moves rather than in a constant posture, how the object looks from the camera is constantly changing. Since the installation site and the operation details of the robot were unknown in advance, we have devised a way to flexibly set the detection range according to the camera installation position and how Ryosan’s demonstration is conducted at the actual demonstration. In addition, if the detection sensitivity is too high or too low, the system itself is useless. We have devised a way to adjust the sensitivity properly when comparing with past images by creating multiple parameters.
3) AI recognition model compression
The original AI recognition model was developed on the assumption that it runs on the GPU. While GPUs are powerful, they are also expensive. In order to provide this demo system to many customers, it needs to be inexpensive. Therefore, this time we chose Raspberry Pi instead of GPU. However, since Raspberry Pi does not have as much performance as GPU, we have devised a way to compress the AI recognition model.
Demand for collaborative robots is growing to solve social problems such as the spread of infectious diseases and labor shortages, and it is predicted that the global market will grow at an annual rate of 40% or more. We will strive to focus on the development of AI for robots including robotic vehicles and contribute to solving social problems.